In this series on how to understand a shop floor, I talked a lot about the physical shop floor—which in my view is the more important part. However, as mentioned in my last post, looking at already collected data also gives a lot of insight into the shop floor. Depending on the aspect you are interested in, data may be the only way to grasp the situation. Hence, this post will look deeper into how to work with data to understand the shop floor.
Introduction
As mentioned in my previous post, using collected data can have a few advantages:
- The collection of data can be outsourced.
- It’s easier to see historical trends.
- It’s usually numerical, which makes subsequent analyses and calculations easier.
- You can track hard-to-see events like infrequent quality mistakes.
On the other hand, data has also a few disadvantages:
- It is merely a representation of reality, but this may be flawed (unintentional or intentional).
- It shows you only the data and nothing else beyond that. More effort is needed for root cause analysis.
- There may be more delay between observation, analysis, and countermeasures.
Hence, using data requires a bit of precaution.
Do You Understand the Data?
The first step when looking at data is to actually understand the data. What does the data actually mean? That sounds easy, but it is not. All the time, I see people make assumptions that turn out to be wrong.
For example, I had a case where we needed the cycle times for a set of injection molding machines. Luckily, there were plenty of data sheets for the different machines and products giving us the cycle times. Unfortunately, they used a different definition of cycle time. For me, the cycle time is the best repeatable time between the production of one part. In other words, if there are no losses, the OEE would be the cycle time… which usually happens only for a few parts before random losses turn your cycle time into an average takt time. However, the maker of the data sheet of the injection molding defined cycle times differently, where the cycle time was merely the time needed to inject the soft plastic into the mold. It did NOT include cooling time, opening the mold, removing the part, and closing the mold again. This is also a possible definition of cycle time, but VERY different from what we needed and expected.
Or another example is statistics on what percentage of the time a machine is stopped. Here, too, the systems of the different machines delivered data on when and how long each machine was in which state. Unfortunately, the definition of “stopped” varied widely among the different machines, sometimes even from the same maker. The definition of a machine being stopped could or could not include any of the following like “lack of material,” “breakdown,” “blocked,” “generally idle,” “maintenance,” “repair,” and many more. Therefore, it was pretty much impossible to compare the data of the different machines due to the widely different definitions.
This gets even worse for aggregated data. For example, I have a whole blog post on how to fudge the OEE. Depending on how it is calculated, it may or may not include breaks, lack of material, or maintenance. I have even seen a generous lump-sum sixty minutes of maintenance included every day, even though the actual maintenance barely lasted five minutes. And, yes, the different definitions of takt time also significantly change your result. This makes it also very hard to compare the OEE‘s of different machines even within the same plant, let alone other plants or even other companies.
Another example for a commonly fudged aggregated measure is the delivery performance. Depending on which plant or company you are in, the definition of “on time in full” (OTIF) can also vary widely. Hence, if you want to use the data, you first have to understand the data. Any false assumptions and your actions are based on flawed data. Or in short, garbage in, garbage out.
Do You Trust the Data?
The next question is, do you trust the data? Data can be wrong, either intentionally or unintentionally.
I once was involved in setting up a warehouse. To estimate the space needs, we needed to know which material came in what type of package. Is is a pallet, a pallet cage, one of the standard industry plastic boxes (which size), etc.? Luckily, our SAP system had the corresponding data for all materials. Unluckily, this was a required field for data entry, and since the data entry guys did not know it either, they just filled in whatever. As a result, the data was unusable. Engine blocks supposedly came in the smallest plastic box, whereas O rings were supposedly delivered on pallets.
Data can also be wrong by simply being out of date. It takes a LOT of effort to keep all data up to date. Probably among the best are automotive and aircraft makers… but a car maker spends in average around EUR 60 000 just to manage a simple part number. Not to design or buy, merely to keep the data current. Multiply this by thousands of part numbers and we are quickly talking real money. A maker of washing machines was less stringent, and spent only 10 000 EUR per part number. This is still a LOT of money, but cheaper than for automotive. On the other hand, you will have problems with wrong and outdated data.
Using the cycle time example from above, these are also metrics that change over time, and a three-year-old cycle time is probably dubious and should be checked. Many data entries are out of date after a few years due to the continuous improvement process.
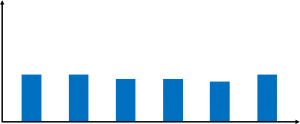
Finally, besides such mistakes and outdated data, there is also intentional manipulation. Data is often uses to determine the performance based pay and the promotions of managers. Unfortunately, it is often easier to manipulate the data than to actually improve the shop floor. For example, one company measured the inventory level on a monthly basis, always at the end of the month. It looked like the graph on the left.
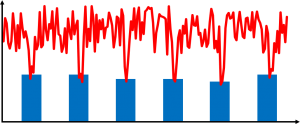
At one point they decided to try to measure the inventory levels on a daily basis. Not all inventories but way too many showed a curve similar to the graph on the right. Miraculously, the inventory levels always dropped significantly at the end of the month. Again, was this coincidence, or was this an intentional effort to fudge the numbers? (Hint: It was not a coincidence!). I have a whole small series of blog posts on Lies, Damned Lies, and KPI.
Do You Clean the Data?
Once you understand and trust the data, you probably need to clean it. Sometimes you may be lucky and the data is usable for further analysis right away. But in many cases, you need to clean the data. This is a crucial step in the data analysis process. It involves identifying and correcting (or removing) errors and inconsistencies in data to improve its quality and ensure that it is suitable for analysis.
You may have to remove duplicate or corrupted entries, add missing values, correct typos. Excel is famous for auto correcting, and scientists renamed twenty-sevn human genes simply because Excel always interpreted their names like “March1” as dates. That different parts of the world use different decimal separators does not help either (10.021 in English countries is 10,021 in many others, where 10.021 in the other countries would be 10 021 in English). And don’t get me started on non-metric units.
Outliers may have to be identified and corrected, different data sources consolidated, and generally the data to be organized in a consistent and useful way. This can take a lot of time, sometimes more than the collection and analysis combined. But skipping this step again leads to garbage in, garbage out.
Only when you have understood the data, believe it, and have it cleaned up can you finally start to analyze the data. With a bit of luck, you may even get to the point where you improve something on the shop floor with the help of the data. Now, go out, understand, verify, and clean your data to understand your shop floor, and organize your industry!
Hi Christoph…Some key fundamentals here which are often overlooked. Good series.
Lies, damned lies and statistics, eh?!
Steve: Yup, exactly!